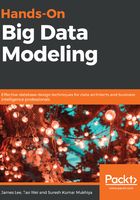
上QQ阅读APP看书,第一时间看更新
Challenges of big data
There are certain key aspects that make the big data very challenging. In this section, we'll discuss some of them:
- Heterogeneity: There is a great deal of diversity in the information consumed by human beings, and they are indeed tolerated as well. In fact, the nuance and richness of natural language will provide valuable depth. However, machine-analysis algorithms expect consistent knowledge, and can't understand nuance. As a consequence, knowledge must be carefully structured as a first step to (or prior to) knowledge analysis. Computer systems work most efficiently if they can store multiple things that are all identical in size and structure. Economical representation, access, and the analysis of semi-structured knowledge require further work.
- Personal privacy: There is a lot of personal information that is captured, stored, analyzed, and processed through internet service providers (ISPs), mobile networks, operators, supermarkets, local transportation, educational institutions, and medical and financial service organizations, including hospitals, banks, insurance companies, and credit card agencies. A great deal of information is being stored on social networks such as Facebook, YouTube, and Google. This illuminates that privacy is an issue whose importance, particularly to the customer, is growing as the value of big data becomes more apparent. This personal data is used by mining algorithms to personalize news content and to manage ads, and for other e-commerce advantages. This is clearly a violation of personal privacy.
- Scale: As the name suggests, big data is massive. When there is an increase in size, there are underlying issues that accompany it in terms of storage, retrieval, processing, transformation, and analysis. As mentioned in the introduction, data volume is scaling much faster than computer resources and CPU speeds, which are static.
- Timeliness: This is concerned with speed, as the larger the size of the data to be processed, the longer it will take to analyze it. There are many scenarios where in the results of the analysis are required in real-time or immediately. This creates an extra challenge when building a system that can process the big data in a timely manner.
- Securing big data: Security is also a big concern for both enterprises and individuals. Big data stores can be engaging targets for hackers or complex persistent threats. Security is an essential attribute in the big data architecture that reveals ways to store and provide access to information securely.